Predicting fallen angels in finance with quantum computers
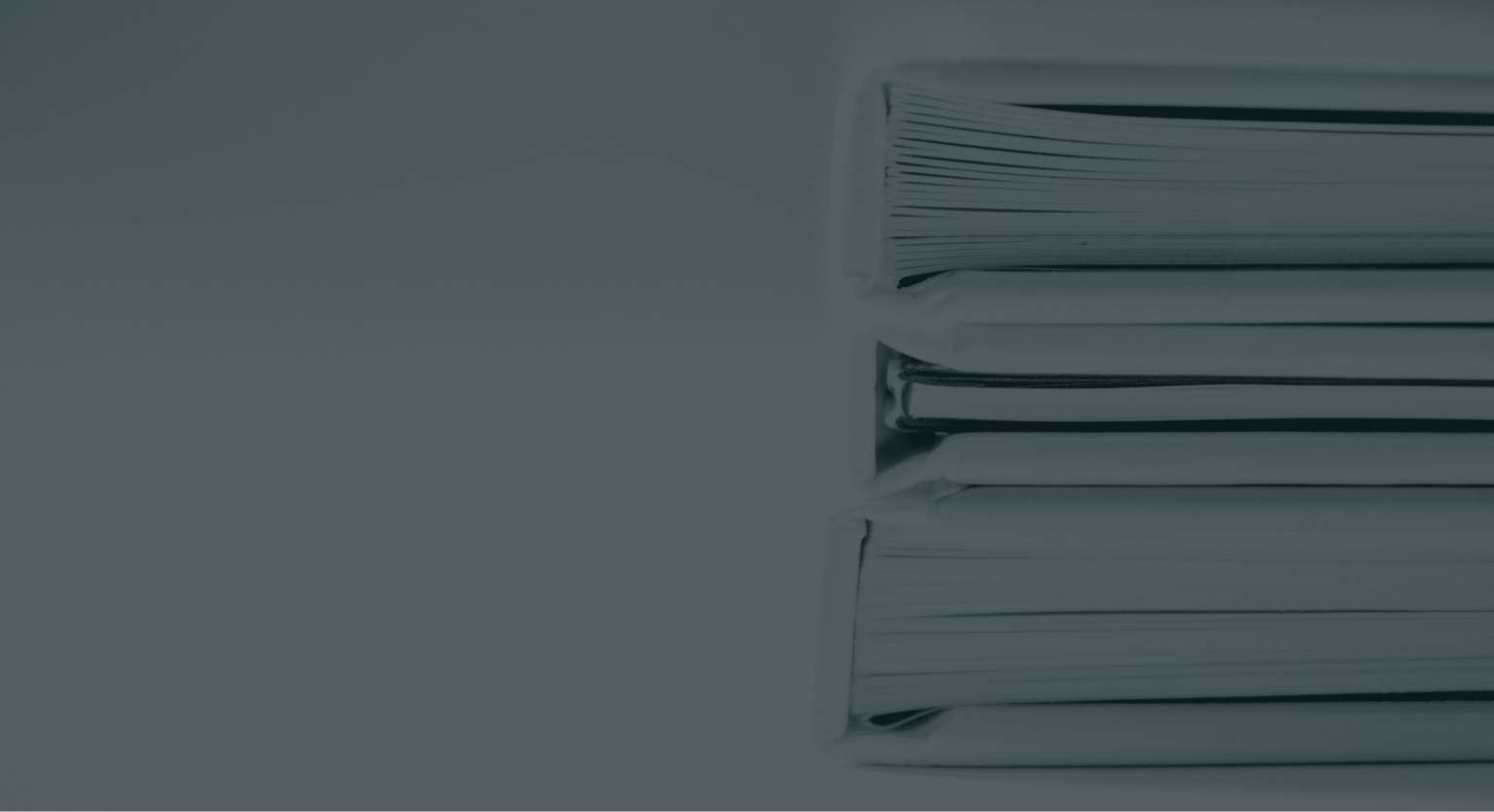
Predicting fallen angels in finance with quantum computers
By pulling spending forward, credit is one of the economy’s most important drivers—much of what people regard as money is, in fact, credit…
Credit ratings and fallen angels
By pulling spending forward, credit is one of the economy’s most important drivers—much of what people regard as money is, in fact, credit. For instance, about 50 trillion of the US economy is credit, dwarfing the 3 trillion in actual money. Thus, the economy’s healthy functioning hinges upon lenders’ ability to accurately assess borrowers’ ability to repay. Let’s not forget that the 2008 financial crisis was partially driven by an increase in the number of borrowers unable to repay their loans.
There are industry-wide standards to assess the risk of lending to a specific entity, whether it is a government or a company. Borrowers are classified by rating agencies like Standard & Poor’s, Moody’s, or Fitch based on a wide variety of data—financial statements, industry analysis, etc. Their credit-worthiness is expressed using a letter-based system going from the safest ‘AAA’, to those more likely to default ‘D.’
Credit ratings are not static. At any given time, a ‘AAA’ borrower’s financial condition might deteriorate and cause a downgrade. In some cases, a borrower can cross the boundary between ‘investment grade’ (BBB- or safer) to ‘high yield (BB+ or riskier). Borrowers facing this predicament are known in the financial industry as ‘Fallen Angels.’
Predicting the likelihood of an angel fall can be vital for debt holders. Failing to predict such events costs lenders several billions per year in missed revenue opportunities. Having a reliable early warning system could guide the implementation of proactive measures to manage changes induced by fallen angels in their portfolios’ risk/return profile.
But this is easier said than done. Fallen angel prediction is a complex computational problem that calls for radically new approaches. That is why we joined forces with the corporate and investment banking arm of Crédit Agricole Group (CACIB) and Multiverse Computing to explore how quantum techniques can help make headway in this critical problem.
CACIB’s random forest model
Today, CACIB addresses this question using a state-of-the-art machine learning model. This model has been trained on a comprehensive dataset collected by CACIB for over 20 years, from 2001 to 2020. The dataset contains information from 2300 companies from 10 different industrial fields and 70 countries. It comprises a total of 91000 instances, one of the largest data sets ever examined, characterized by 153 features, and each record is labelled by whether it is a fallen angel or not.
CACIB relies on a popular machine-learning technique known as a ‘random forest.’ Random forests rely on classifiers known as ‘decision trees.’ These functions take in a company’s features and decide whether it is a fallen angel. For instance, you could plug in the company’s liabilities, country of origin, industry, etc., and the tree tells you whether it is a fallen angel. A random forest combines the results of several decision trees to build a consensus prediction. Random forests are an instance of an ensemble method, where the insights derived from various classifiers are combined to improve predictive power.
CACIB’s random forest model displays a sensitivity of 83% and a precision of 28% in production. Clearly, there is room for improvement! One could simply increase the number of variables or the dataset size, but the model scales poorly and requires an inordinate amount of computational resources. Could quantum capabilities help?
Enhanced prediction with neutral-atom quantum processors
We introduce a new type of ensemble method to classify fallen angels. As mentioned above, these methods involve reaching a consensus between the predictions of various classifiers. The key question is: should we take every prediction equally seriously or assign different weights to each classifier? The latter turns out to be the right approach. Training the individual classifiers is something classical computers can handle efficiently. However, finding the optimal weights is immensely complicated for them.
This is where PASQAL’s quantum processors come in handy.
Our processors display unprecedented scalability and are capable of generating complex entangled states. These features give our machines the ability to handle complex optimization problems efficiently. In this case, the question of finding the weights that maximize the model’s predictive capabilities within a reasonable timeframe. In a recent paper, we introduced a proprietary algorithm known as Random Graph Sampling designed to achieve this feat.
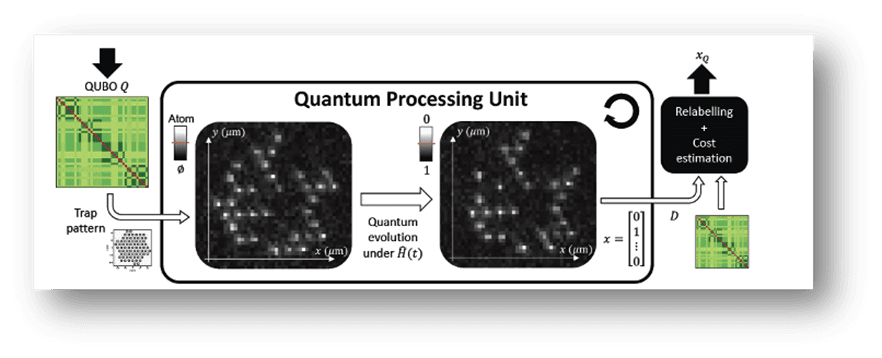
We tested our method as part of an end-to-end workflow—from training to prediction—exploiting real quantum hardware from PASQAL. We matched the performance of CACIB’s highly-optimized machine learning method using 96% fewer classifiers. A much smaller forest, if you will. Interestingly, we achieved this with just 50 qubits.
This is the first time a quantum-powered workflow state-of-the-art classical approach achieves this. As we increase qubit count and accelerate the operation speed of our quantum processors, we expect radical improvements. Indeed, relying on Multiverse’s software we simulated how this approach could scale to our upcoming quantum processors. We expect to be able to consistently beat the classical benchmark by 2024, making the approach production-ready.
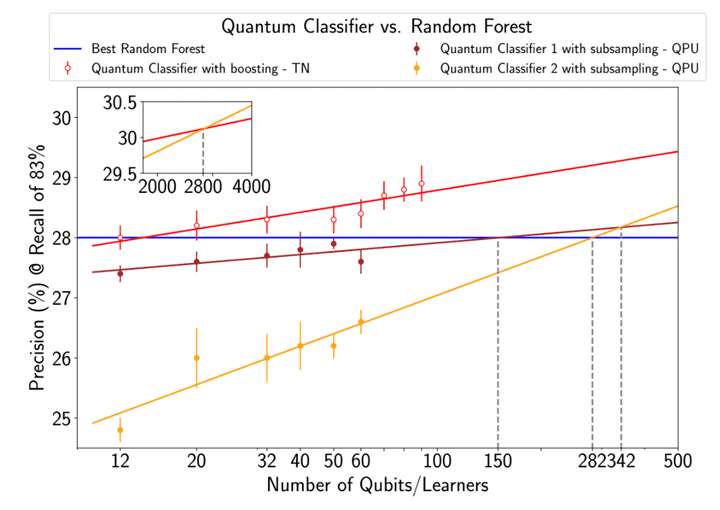
Outlook
The financial industry has always been an early adopter of novel computational techniques, from double-entry accounting in the Renaissance to quantitative trading. This collaboration shows that quantum computing will not be the exception.
Original publication
You can read here our original publication on Arxiv